Clinicians, computer scientists, and ethicists are working across the University of Rochester to incorporate reliable and ethical AI into medical diagnosis and treatment.
In 2012, professor of psychiatry Caroline Easton had a eureka moment watching her son play video games: what if she could blend video-game technology with her research on the intertwined issues of addiction and domestic violence?
“Younger clients have grown up with games and having technology at their fingertips,” she says. “We need to have venues of therapy that are relatable to them and that standardize behavioral therapy in a way that is like a medication.”
With that insight in mind, Easton set out to develop a platform that would use an avatar coach to guide patients through cognitive behavioral therapy and help them practice coping skills.
Initially, Easton and her team manually created and animated rudimentary avatars. But increasingly sophisticated artificial intelligence (AI) technology has allowed her to fine-tune the tool, enabling users to customize their avatar coaches to respond to their particular needs.
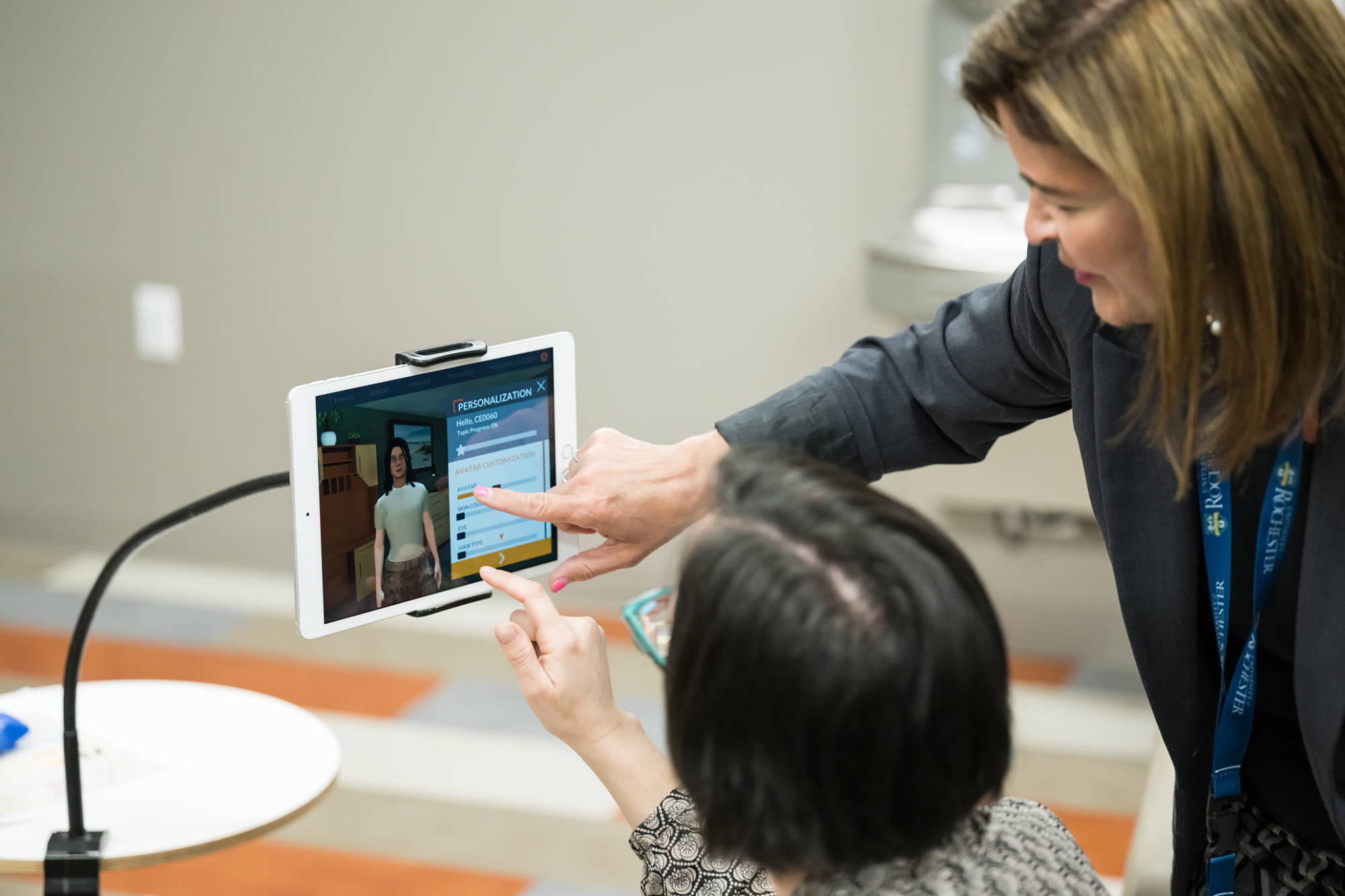
Funded by the National Institutes of Health, the app is in the pilot phase, with randomized control trials set to begin by the end of the summer. Easton says AI tools like this one promise to transform addiction treatment by not only supporting patients between therapy sessions but also enabling clinicians to spend time and energy with their patients in ways that are even more meaningful.
“We can now use AI to collect data and deploy the most relevant coping skills based on what the client is feeling in the moment,” Easton explains. “And if we can also decrease things like compassion fatigue, vicarious trauma, and work burnout—and allow the clinician more time to focus on the therapeutic alliance—I feel like we are heading in the right direction.”
Today, Easton is the Medical Center’s academic chief of addictions psychiatry and director of digital therapeutics for the Department of Psychiatry. Her use of AI in therapies illustrates one facet of AI’s transformative power in medicine and health care.
At the same time, AI also raises questions among clinicians and researchers, including Easton: How can we ensure everyone benefits from AI advancements fairly and responsibly? As AI takes on a larger role in health care, how do we guarantee it serves everyone’s best interests? How can advanced technology be integrated into medicine without losing the human connection?
The long road to generative AI
Artificial intelligence is not new. It was described in theory as early as the 1950s by philosophers and mathematicians such as Alan Turing, who posited that machines might be able to learn in ways similar to humans. But it took decades before computing power was sufficient to make AI more than just a concept.
In the late 1990s, IBM demonstrated a major breakthrough when its Deep Blue computer defeated world chess champion Garry Kasparov. Then in 2011 a still more powerful IBM computer system called Watson competed on the TV game show Jeopardy! and won over two of the game’s greatest champions, Brad Rutter and Ken Jennings.
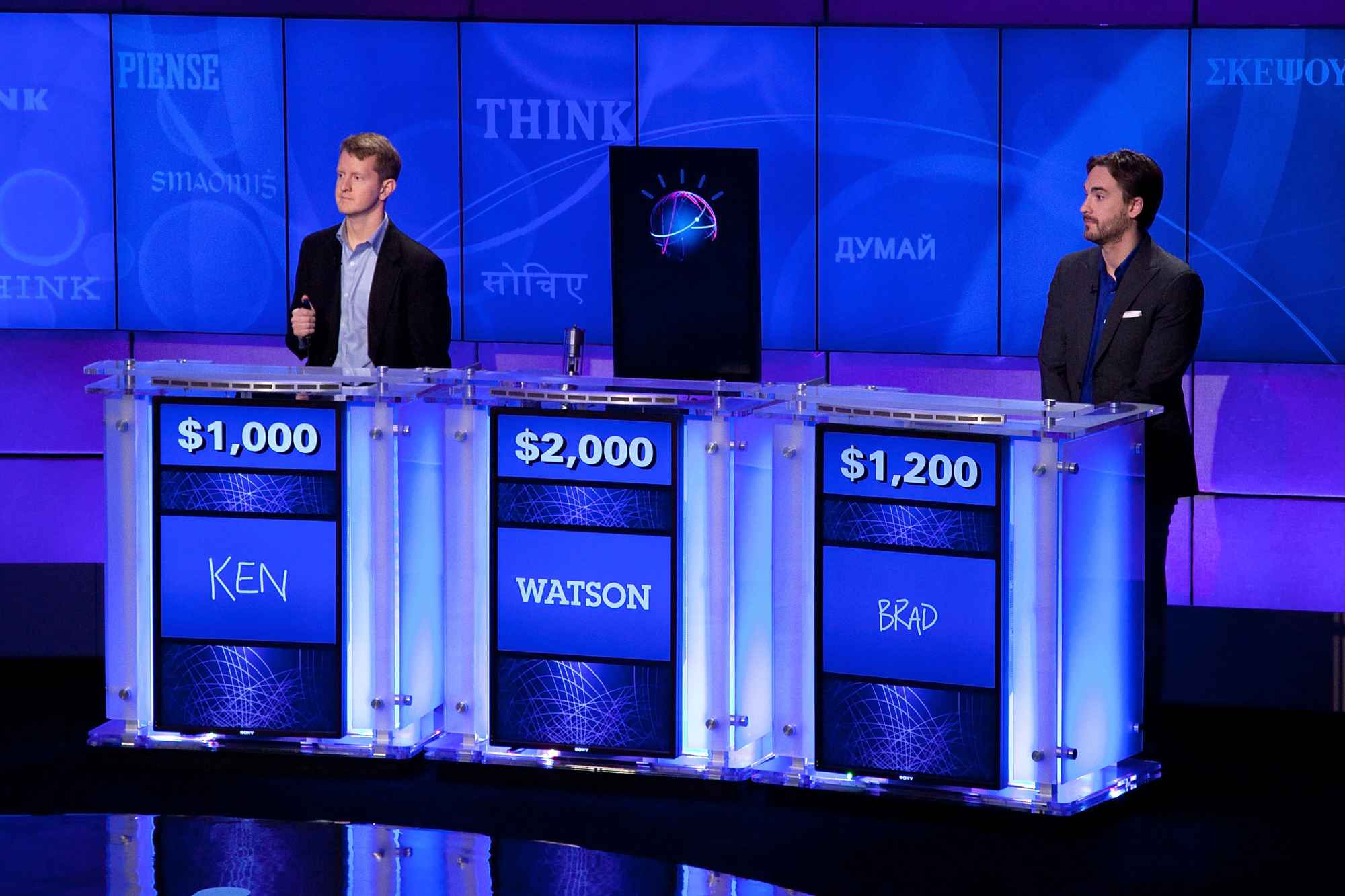
When IBM tried to leverage Watson in the health care sector, however, the effort was unsuccessful. Watson was initially trained on highly structured datasets, such as dictionaries and encyclopedias. That training was poorly suited to working with health care data. Inherently unstructured, health care data encompasses a wide range of complexities, including shorthand and misspellings in doctors’ notes; variability in the image quality of medical scans; the presence of anomalies such as rare diseases and complex genomic information; and fragmented datasets spread across different systems. Computers required sophisticated techniques to effectively process and integrate diverse types of data.
In 2023, a paradigm shift occurred with the emergence of generative AI, led by OpenAI’s ChatGPT.
“When the world got introduced to generative AI, in health care—and pretty much every aspect of our daily lives—the potential of AI became very powerful,” says Michael Hasselberg ’13N (PhD) an associate professor of psychiatry, clinical nursing, and data science, and the University of Rochester’s inaugural chief digital health officer.
While classical machine learning enables computers to analyze data and use it to make predictions, generative AI enables machines to create new content based on learned patterns from large datasets. It does this using complex neural networks designed to mimic the human brain’s ability to recognize patterns and learn from them.
“Generative AI comes trained on more than a trillion parameters—essentially the entire internet and all of its structured and unstructured data,” Hasselberg explains.
AI’s advantages
That powerful foundation allows generative AI to analyze patterns in medical images with remarkable accuracy and speed.
“AI helps us identify urgent issues quickly, which is crucial for conditions like a pulmonary embolism or a brain bleed,” says Jennifer Harvey, the Dr. Stanley M. Rogoff and Dr. Raymond Gramiak Professor in Radiology and chair of the Department of Imaging Sciences.
That means AI tools can act like a second set of eyes for radiologists. Say a patient undergoes a CT scan to check for possible pneumonia. As the scanner captures detailed images of the patient’s chest, an AI algorithm analyzes each of the images, and, within moments, flags a potential pulmonary embolism even if the scan was initially done for a different reason. Potentially urgent problems get prioritized, leading to more efficient and accurate diagnostics.
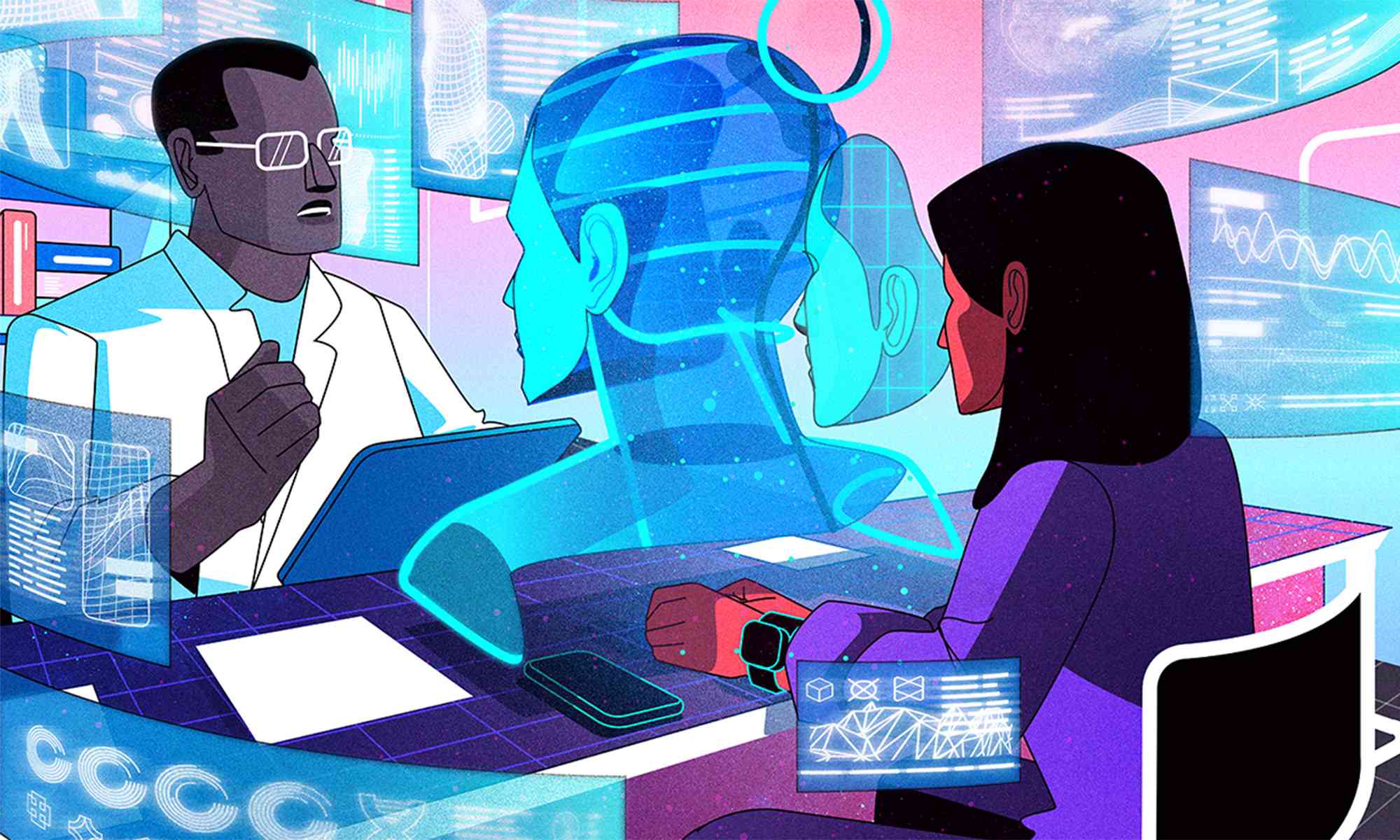
But radiologists must still carefully examine every patient scan. According to Harvey, AI does not threaten to replace radiologists because most algorithms are built to identify a single finding. For instance, the tool might only be looking for a pulmonary embolism in a set of images. Rare findings continue to be difficult for AI tools to detect.
“Radiologists are still much better at synthesizing the findings in a way that AI tools cannot,” Harvey says. “For example, a chest CT may have one or two findings flagged by AI, but the radiologist must put all of the findings together to generate likely diagnoses.”
Still, she adds, predictive AI tools can offer “critical insights”—and not only in analyzing scans. They can also summarize report results and automate other clinical tasks.
Hasselberg highlights the “army of nurses” at the Medical Center whose jobs previously involved manually extracting data points from patient charts for submission to national registries.
“Tasks like these are well below the scope and practice of a nurse,” he says. “You’re still going to have a human in the loop that will look at the generative AI’s output and see if everything looks right. But the machines take the administrative burden off the clinicians, giving them more time to spend with patients actually doing clinical care.”
Can doctors and patients trust AI?
To rely on algorithms for disease detection and treatment, however, clinicians need to have high confidence in their accuracy. Says Hasselberg: “There is some risk with generative AI because it does generate new content. It can get it wrong.”
Hasselberg’s role includes serving as codirector of the UR Health Lab, using technology such as machine learning, virtual reality, and 3D imaging to enhance patient care, while also tackling ethical challenges. To this end, he has partnered with stakeholders across the University, including not only clinicians like Harvey and Easton but also Chris Kanan, an associate professor of computer science who helped develop the first FDA–cleared pathology tools, and Jonathan Herington, an assistant professor of philosophy and of bioethics who is a nationally recognized expert on the ethical issues surrounding AI.
An ethicist whose research once focused on political philosophy, Herington began writing about AI systems in 2017, prompted by articles in the popular press on the use of machine learning algorithms to predict criminal recidivism. He began to look deeply into how algorithms can perpetuate social and cultural biases.
From 2021 to 2023 he served on the AI Task Force for the Society for Nuclear Medicine and Molecular Imaging. During that time, the task force published two influential papers in the Journal of Nuclear Medicine addressing ethical issues surrounding AI.
Herington highlights one case demonstrating a particularly pressing ethical concern: An insurance algorithm was designed to identify patients who have a high probability of requiring frequent care, with the goal of enrolling them in prevention programs. As a metric for identifying high-need patients, the insurer used cost of care—that is, “How much care did a person cost us last year?” Due to disparities in access, Black patients who were as sick as white patients were rated with lower risk because they had historically accessed care less frequently.
“All of this historic bias in the health care system got baked into this dataset,” Herington says.
One way to remediate bias is to be more deliberate about the data used to train the system. But a larger issue is at play as well. Precisely because the tools can get it wrong, “it is more important than ever—especially as we start moving toward more generative AI tools—to always have a human in the loop,” Herington says.
Perfecting AI tools
Another way to keep humans in the loop is to regulate AI tools as medical devices, which requires FDA compliance certification. Herington favors that approach. “Right now, it’s like the Wild West,” he says. “Models can be implemented without establishing that they work.”
Many companies balk at the rigorous testing—and the delay that ensues—in moving a product to market. However, FDA certification offers benefits even beyond ensuring a product is safe. The distinction helps increase the trust hospitals and clinics have in a product, Kanan says. If a product does not go through the FDA certification process, it must be marketed in the US as “research use only.” FDA distinction also ensures regulatory compliance to avoid legal issues and is more likely to be covered by insurance.
The FDA process proved valuable when Kanan was working with the company Paige.AI to develop Paige Prostate—the first FDA–cleared, AI-assisted pathology tool.
In his lab, Kanan focuses on fundamental research in deep learning as it applies to AI.
His work with Paige began in 2018, with the goal of developing an AI tool that could assist pathologists in diagnosing cancer with unprecedented speed and accuracy. To create such a tool, however, required more than developing sophisticated algorithms. It also meant integrating AI into workflows and ensuring its reliability across various settings.
Kanan and his team had to demonstrate the AI could perform consistently well, regardless of differences in medical data and environments of individual hospitals. For instance, an algorithm trained on data from a specific set of hospitals might be more sensitive to the unique conditions and nuances of those environments. When the same algorithm is then applied to a different patient population or hospital system, it can struggle to perform effectively because of variability in data and clinical practices. Kanan’s team had to ensure Paige Prostate wasn’t overly sensitive to factors such as the type of microscope used or the presence of watermarks on medical scans. They also needed to address potential biases related to patient demographics, such as gender or race, by ensuring Paige Prostate performed equally well across hospitals serving different populations.
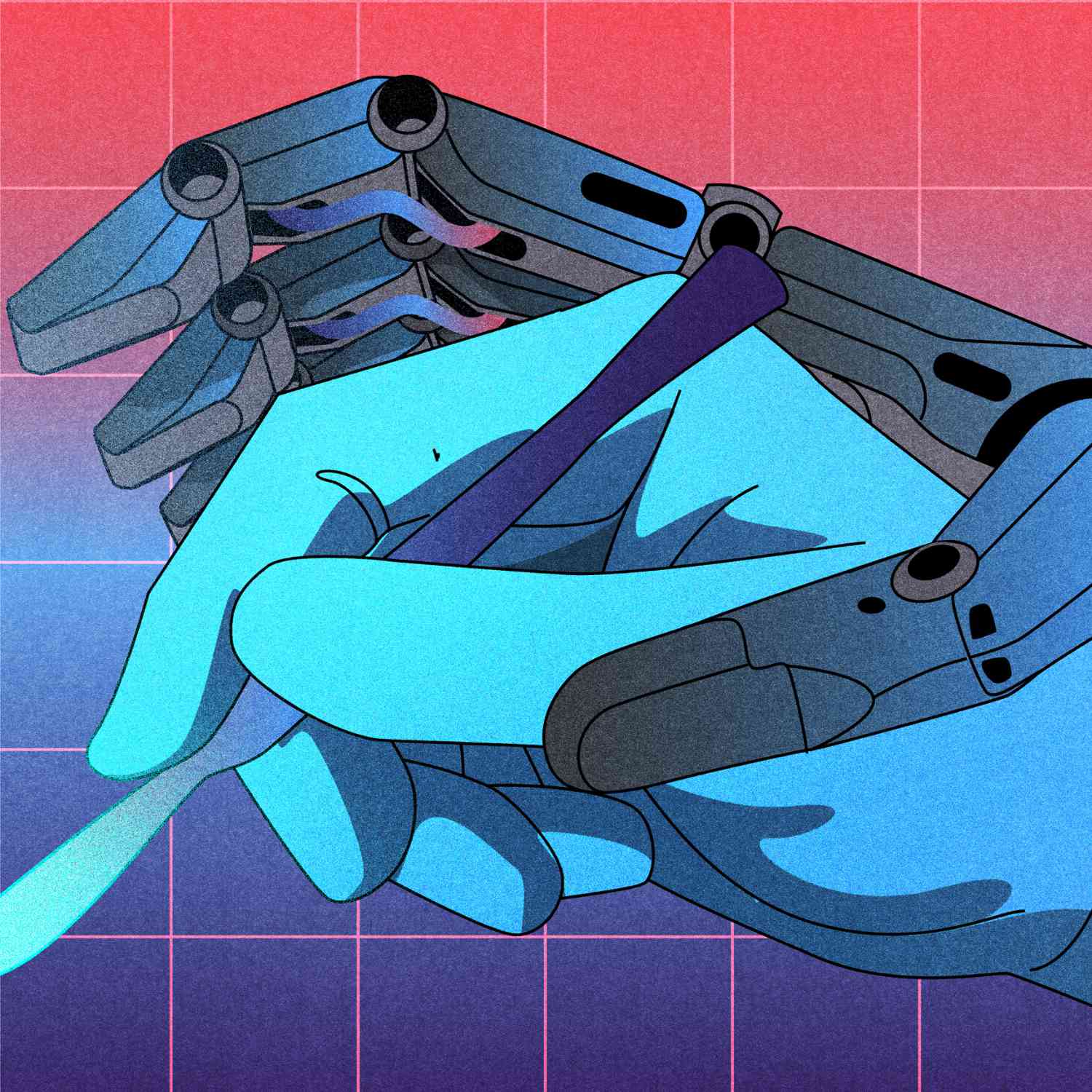
Then there was the challenge of making sure the AI could detect a variety of anomalies.
“The majority of the cases are the ones that are obvious,” Kanan says. “It’s the rarer stuff that people don’t have as much familiarity with that are challenging for an AI system to spot.”
The key, Kanan says, is to train AI systems on extensive and diverse datasets. In Paige’s case, the dataset included millions of images and datapoints from numerous sources, ensuring the tool could generalize well across different conditions.
It’s also important that system training be continuous. This is where academic medical centers can play a large role. By implementing AI programs and contributing valuable data, hospitals can work with companies like Paige to refine and enhance AI algorithms. This collaboration ensures that AI tools continuously “learn” and improve.
Locations such as Rochester—which cover broad, diverse patient groups, and, in Rochester’s case, also span dense urban areas as well as small towns and rural communities—are well positioned for the task of testing and refining AI tools.
“Having that heterogeneity of patients is a game changer,” Hasselberg says. “It’s incredibly important to make sure that AI tools are developed and deployed in an ethical way that accounts for a very diverse group of patient populations.”
What no machine can do
Despite the technological advancements in AI, the role of human expertise and empathy remains irreplaceable. Generative AI, even at its most impressive, “doesn’t correlate with the ability to plan or have beliefs, attitudes, or genuine emotional reactions,” Herington says.
Says Hasselberg, “There’s an art to clinical care and the way we make decisions. It’s not all based on data points or an algorithm; it’s based on intuition and experience. There are things we do as clinicians that a machine can’t do.”
That’s precisely why Easton designed her app to be a complement to—not a replacement for—clinician-centered therapy.
The app’s program spans 12 weeks—a length of time consistent with a typical course of cognitive behavioral therapy. Patients start each session by rating their cravings, substance use, and mood in the current moment. The avatar then guides them through real-time coping exercises and positive reinforcements, such as meditations or distraction techniques like taking a walk. Patients provide feedback by rating the effectiveness of the exercises. The tool tracks progress and behaviors with charts and graphs, identifying patterns in each patient’s stressors over time.
Easton envisions that the program could be integrated into a wearable device to take in biomarker data and proactively deploy on-demand coping skills to help prevent psychiatric crises.
But this would be a tool that patients would use in between visits with a human clinician, who could synthesize the information and integrate it into a detailed care plan.
It is never the goal, Easton says, for technology to replace the human therapist.
“We should never be taking the human out of the equation. We can coexist and integrate with technology, but I don’t envision a world where technology would ever take over for people.”
This story appears in the summer 2024 issue of Rochester Review, the magazine of the University of Rochester.